Companies need a crystal ball to make informed decisions in today’s complex, dynamic, and unpredictable business landscape. That’s where forecasting models emerge – the unsung heroes of strategic planning and project management that bridge current decisions with future outcomes.
Qualitative and quantitative forecasting models are potent tools that allow businesses to anticipate trends before they emerge, identify potential risks early on, and exploit opportunities before the competition does.
This guide is your one-stop shop for unlocking the power of forecasting models. We’ll cover different types of models, from qualitative to quantitative ones, and explore the art and science behind selecting and deploying the suitable model for your business or project.
So join us as we uncover the application of forecasting models in business strategy – an invaluable tool that can make all the difference on your company’s path to success! It’s time to peer into the future.
Throughout this article, we’ll discuss:
- The Essence of Forecasting Models: What Are They?
- Types of Forecasting Models
- 4 Widely-used Qualitative Forecasting Models
- 3 Highly Effective Quantitative Forecasting Models
- Wrap-up: Choose the Right Forecasting Model for Business Success
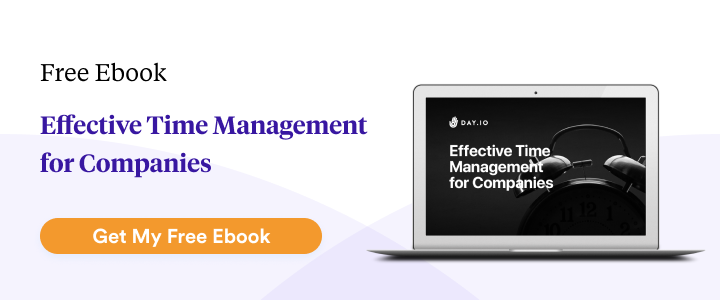
The Essence of Forecasting Models: What Are They?
Forecasting models are mathematical tools used to estimate future values of a particular variable, such as sales or revenue. They provide a way for businesses to predict what might happen and plan accordingly. Models vary from simple to complex; some use historical data, while others consider external factors like economic conditions or customer behavior patterns.
The goal is to help companies make informed decisions affecting their long-term success by providing an accurate outlook on likely market trends and events. By combining forecasting with current data, executives can identify potential risks early on and develop strategies that will best position them for success in the future.
Types of Forecasting Models
Businesses typically use two broad types of forecasting models: qualitative and quantitative. Here’s what they mean:
Qualitative Models
Qualitative models are subjective and rely on personal opinions, expert judgment, or the collective wisdom of a group. They generally use qualitative data like customer feedback surveys or market research to predict future outcomes. These models are mainly used for short-term forecasting because they don’t consider external factors such as economic conditions or political events. Some examples of qualitative forecasting methods include:
- Delphi method
- Market research
- Visionary forecast
- Sales force composite
Qualitative models are best for scenarios with no available data or when a business needs to decide quickly. However, they can be less accurate than quantitative models and may not provide adequate detail.
Quantitative Models
Quantitative models use historical data and mathematical equations to generate forecasts. These models can be used for long-term forecasting because they consider external factors like economic conditions or customer behavior patterns. Some examples of quantitative forecasting methods include:
- Time series analysis
- Regression analysis
- Econometric models
Quantitative models offer more accuracy and detail than qualitative models, making them ideal for long-term forecasting. However, they require vast amounts of data and can be time-consuming to build.
We’ll examine a few popular qualitative and quantitative models in more detail.
4 Widely-used Qualitative Forecasting Models
Now we’ll explore qualitative forecasting models. These are based on judgment and expert opinion rather than past data and statistical analysis. They can help make decisions when little or no historical information is available.
-
Delphi Method
The Delphi method is a consensus-based forecasting technique. It involves gathering opinions from experts on the subject and synthesizing them into a single forecast. This approach relies on group feedback to reach an agreement that can be used for decision-making purposes.
For example, a restaurant chain can use the Delphi method to forecast customer demand and sales by gathering opinions from restaurant managers, chefs, servers, etc.
Pros
The Delphi method is excellent for gathering opinions from a wide range of experts, which can provide a much more accurate forecast. It also helps reduce the risk of bias when relying on one individual’s opinion.
Cons
This approach can be pretty time-consuming and expensive if too many people are involved in the process. Additionally, reaching a consensus among all participants can be difficult due to conflicting views or interests.
-
Market Research
Market research involves gathering and analyzing data from customers, competitors, and other industry stakeholders to understand the market dynamics. Market research can be used to identify trends and forecast future scenarios.
For example, a retailer can use market research to anticipate customer demand for specific products or services. By understanding consumer needs and preferences through surveys or focus groups, the company can gauge what products will likely sell shortly.
Pros
Market research provides insights into customer behavior that other forecasting methods may not capture. It also allows companies to gain a better understanding of their competitive environment.
Cons
Market research requires much time and resources to collect and analyze data. Additionally, the accuracy of the results can be affected by biases in the sample population or changes in market conditions.
-
Visionary Forecast
The visionary forecast is based on the opinion of a single individual or group. This approach relies heavily on intuition and experience rather than data analysis. It is often used when there is limited or no historical information for the forecasted situation.
An aerospace company may use this approach to predict future trends in their industry by gathering insights from engineers and other experts in the field.
Pros
The main advantage of using this approach is that it can provide valuable insights into future trends that would otherwise be difficult to obtain through traditional methods such as data analysis. Additionally, it can be less time-consuming and cheaper than other methods.
Cons
The main disadvantage of this approach is that it can be prone to bias due to the subjective nature of the opinions being gathered. It may also not provide as accurate a forecast as quantitative methods.
-
Sales Force Composite
The sales force composite is a qualitative forecasting method based on a company’s salespeople’s opinions. This approach relies on their experience and knowledge to forecast future demand for products or services.
For example, a clothing retailer can use this approach to estimate demand for its new line of winter jackets by gathering input from store managers and other sales personnel.
Pros
The primary benefit of using a sales force composite is that it leverages the expertise and experience of those closest to customers. This can provide a more accurate forecast, as they will understand customer needs and preferences in-depth. Furthermore, this method is quick and cost-effective compared to alternative approaches.
Cons
Unfortunately, the downside of this approach is its reliance on subjective opinions, which could lead to inaccurate predictions. Moreover, salespeople may have conflicting interests when supplying information because their performance is linked with sales figures.
3 Highly Effective Quantitative Forecasting Models
Now we’ll move on to the quantitative forecasting models. These are based on historical data and statistical analysis rather than expert opinion or judgment.
-
Time Series Analysis
One of the most popular quantitative forecasting methods is time series analysis. This approach uses past data points to forecast future trends, such as sales or customer demand.
Many businesses use time series analysis to predict future sales and adjust their strategies accordingly. This approach can be used in various industries, such as retail, finance, and manufacturing.
Pros
Time series analysis is relatively easy to implement and can provide more accurate predictions than other methods. Additionally, it requires minimal effort once the initial data points are gathered.
Cons
Time series rely heavily on past data points that may not reflect future trends accurately. Additionally, if there is too much noise or volatility in the data, the accuracy of predictions may suffer significantly.
-
Regression Analysis
Regression analysis uses data points to determine the relationship between two or more variables, such as sales and customer demand. The approach is beneficial when predicting the impact of a particular factor on another.
Pros
Regression analysis is relatively easy to understand and implement. Additionally, it can be used in many scenarios to predict future outcomes accurately.
Cons
The success of regression analysis depends on having a large amount of data points, which can be time-consuming and challenging to obtain. Additionally, it is more complex than other forecasting methods and requires more technical knowledge to use effectively.
-
Econometric Models
Econometric models use statistical and economic principles to forecast future trends. They are appropriate for predicting long-term trends, such as GDP growth or inflation.
Pros
Econometric models are more accurate than other quantitative approaches due to their comprehensive analysis of data points. Additionally, they can be used in various scenarios to predict outcomes with high accuracy.
Cons
The main downside of using econometric models is that they require sophisticated technical knowledge and expertise to implement them correctly. Additionally, the results may not always be reliable due to the complexity and variability of the economic environment.
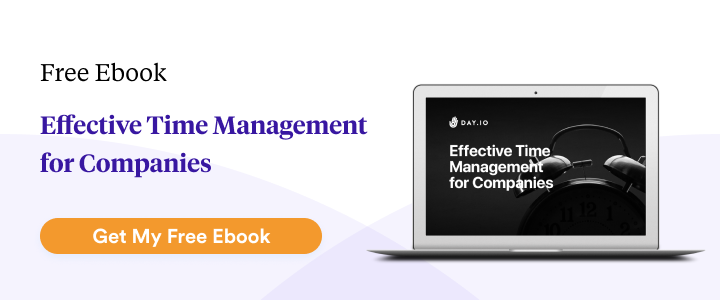
Wrap-up: Choose the Right Forecasting Model for Business Success
Forecasting models can be a powerful compass toward success in pursuing business growth and project management. From the Delphi method to market research or sales composite methods, each model provides insights to help make informed decisions, reduce risks, and capitalize on future opportunities.
But choosing the suitable forecasting model is only half the battle. Tracking time and projects is essential to ensure your forecasts remain accurate and actionable in real time. For this complex task, consider leveraging a robust tool like Day.io to keep your team in sync while giving you access to data that empowers your forecasts with precision.